Designed Experiments: Recent Advances in Methods and Applications
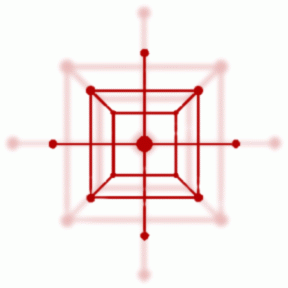
University of Technology Sydney, Australia
14 - 17 December 2015
Programme
Unless otherwise stated, all sessions will take place in the Harris room. Posters, tea, coffee and lunch will be in the bar/foyer area. Registration will be outside the Harris room near the venue entrance.
Each keynote speaker has 40 minutes, plus 5 minutes for questions.
Each invited speaker has 25 minutes, plus 5 minutes for questions.
Each contributed speaker has 15 minutes, plus 3 minutes for questions.
Day 1: Monday 14 December 2015 |
08.30 - 09.45 Registration |
09.45 - 10.00 Welcome |
10.00 - 10.45 Keynote (Chair: Dave Woods) |
Rosemary Bailey (University of St Andrews, UK) "Blocking in multi-stage experiments"
In a multi-stage experiment, the same experimental units are used in each stage but different treatment factors are applied at different stages. Constraints on processing imply that these units must be partitioned into blocks at each stage. However, unlike in the classical situation, the blocks are not inherent, and the designer of the experiment can choose the partition into blocks at each stage. Is it better to align the Stage 2 blocks with the Stage 1 blocks as far as possible or to make them as orthogonal to each other as possible? In either case, how should treatments be assigned? |
10.45 - 11.15 Coffee |
11.15 - 12.15 ISBIS session on discrete choice experiments with applications in industry, business and the environment (Chair: Brad Jones) |
Peter Goos (University of Leuven, Belgium) "Choice experiments involving mixtures"
Many consumer products are mixtures of ingredients. Examples include food products and drinks. For such products, it is generally challenging to determine the ingredient proportions that optimize the consumers' satisfaction with the product. For this reason, many taste experiments exist where respondents have to rank various mixtures from most preferred to least preferred. This approach has various weaknesses. First, the ranks are generally treated as numerical when analyzing the data. Second, respondents often have trouble ranking large numbers of items. We propose the use of choice experiments instead of ranking experiments to overcome these weaknesses. To this end, we embed the traditional Scheffé models for data from mixture experiments in a multinomial logit framework. In addition, we present an algorithm for constructing optimal designs for choice experiments involving mixtures. We use our approach to set up a cocktail tasting experiment, and compare utility-neutral designs, locally optimal designs and Bayesian optimal design for that experiment. To conclude the talk, we discuss extensions of the approach to cope with mixture amounts and with process variables, along with several examples from industry and from transportation. |
Steve Bush (University of Technology Sydney, Australia) "Applications of discrete choice experiments to habitat selection in coral reef fish"
In this presentation we look at the application of mixed logit discrete choice experiments to habitat choice for three species of damselfish found in the Great Barrier Reef on the Queensland coast. In particular, we consider the changes in habitat preference as water temperature changes and briefly discuss the implications for each species should sea temperatures rise. |
12.15 - 12.45 Poster storm (Chair: Ken Russell) |
12.45 - 13.45 Lunch |
13.45 - 14.45 Design for intractable models (Chair: Richard Boys) |
Antony Overstall (University of Glasgow, UK) "Bayesian optimal design for ordinary differential equation models"
Bayesian optimal design requires the minimisation of the expectation (over all unknown and unobserved quantities) of an appropriately chosen loss function. This can be non-trivial due to 1) the high dimensionality of the design space; and 2) the intractability of the expected loss. In this case, a further complication arises from the intractability of the solution to the system of ODEs. We propose a strategy that employs a modification of the continuous coordinate exchange algorithm where a statistical emulator is employed to approximate the expected loss function, and a probabilistic solution to the system of ODEs. The strategy is demonstrated on several illustrative examples from the biological sciences. |
Chris Drovandi (Queensland University of Technology, Australia) "Bayesian experimental design for models with intractable likelihoods using indirect inference"
We present indirect inference as a useful method for Bayesian optimal experimental design for models with intractable likelihoods. The approach estimates the parameters of an auxiliary model with a tractable likelihood in an offline step, using simulations from the generative model, the assumed true model of interest. The current state-of-the-art method to optimise experimental design for models with intractable likelihoods is to use approximate Bayesian computation (ABC). The proposed approach using indirect inference is more flexible, since it is free of the restriction to a discrete design space as in the ABC approach and it is less memory intensive. The use of indirect inference for Bayesian experimental design is illustrated using two stochastic models; a simple death process and an epidemiological model for the evolution of macroparasites, which motivates this work. |
14.45 - 15.30 Posters and Tea |
15.30 - 16.30 New topics in design of experiments (Chair: Rosemary Bailey) |
Haiying Wang (University of New Hampshire) "Optimal designs for big data with linear models"
Although computing resources have been growing rapidly over past decades, their advance still lags far behind the growth of data volume. When the volume of a data set is too large to be fully analyzed with limited computing resources, one way to extract useful information is to reduce the data size by carefully selecting a sub-sample. Existing studies in this direction have been focusing on random sub-sampling from the full data. But this approach brings in random errors and a resultant sub-sample contains information about unknowns at the scale of the sub-sample size, not at the scale of the full sample size. We propose a framework for identifying the most informative sub-samples using optimal experimental design approach. The proposed technique deterministically chooses a sub-sample by maximizing its amount of information about unknowns and it is possible to obtain a sub-sample with a small sample size that preserves information about unknowns at the scale of the full data, i.e., the major information in a massive data set can be extracted by using a small sub-sample. We consider the T-optimality and the D-optimality. For the T-optimality, an explicit solution is available for the optimal design and it is computationally easy to implement. But the T-optimal criterion does not have a statistically meaningful interpretation and it does not always have the best performance. For the D-optimality, the exact optimal design is difficult to obtain, analytically and numerically. We develop an algorithm to approximate the D-optimal design. The proposed approach has similar computing efficiency to the sub-sampling approach, but it is much more efficient in parameter estimation. We obtain the asymptotic properties of resultant estimators and evaluate the practical performance using numerical experiments based on simulated data and real data. |
Tim Waite (University of Manchester, UK) "Random designs for robustness to functional model misspecification"
We consider response surface problems where it is explicitly acknowledged that a linear model approximation differs from the true mean response by the addition of a discrepancy function. The most realistic approaches to this problem develop optimal designs that are robust to discrepancy functions from an infinite-dimensional class of possible functions. Typically it is assumed that the class of possible discrepancies is defined by a bound on either (i) the maximum absolute value, or (ii) the squared integral, of all possible discrepancy functions. Under assumption (ii), minimax prediction error criteria fail to select a finite design. This occurs because all finitely supported deterministic designs have the problem that the maximum, over all possible discrepancy functions, of the integrated mean squared error of prediction (IMSEP) is infinite. We demonstrate a new approach in which finite designs are drawn at random from a highly structured distribution, called a designer, of possible designs. If we also average over the random choice of design, then the maximum IMSEP is finite. We develop a class of designers for which the maximum IMSEP is analytically and computationally tractable. Algorithms for the selection of minimax efficient designers are considered, and the inherent bias-variance trade-off is illustrated. |
Day 2: Tuesday 15 December 2015 |
08.15 - 08.30 Registration |
08.30 - 09.15 Keynote (Chair: Sue Lewis) |
Jeff Wu (Georgia Institute of Technology, USA) "A fresh look at effect aliasing and interactions: some new wine in an old bottle"
Interactions and effect aliasing are among the fundamental concepts in experimental design. Some new insight and approach are given on this time honored subject. Let me start with the very simple two-level fractional factorial designs. Two interactions AB and CD are said to be aliased if both represent and are used to estimate the same effect. In the literature this aliasing is deemed impossible to be “de-aliased” or estimated. We argue that this “impossibility” can indeed be resolved by taking a new approach which consists of reparametrization using the notion of “conditional main effects” (cme’s) and model selection by exploiting the properties between the cme’s and traditional factorial effects. In some sense this is a shocking result as this has been taken for granted since the founding work of Finney (1945). There is a similar surprise for three-level fractional factorial designs. The standard approach is to use simple group theory to decompose the interactions into orthogonal components, each of 2-d. Then the quandary of full aliasing between interaction components remains. Again this can be resolved by using a non-orthogonal decomposition of the four degrees of freedom for AxB interaction using the linear-quadratic parametrization. Then a model search strategy would allow the estimation of some interaction components even for designs of resolution III and IV. Moving from regular to nonregular designs like the Plackett-Burman designs, most of the interactions are not orthogonal to the main effects. The partial aliasing of the effects and their complexity was traditionally viewed as “hazards”. Hamada and Wu (1992) first recognized that this could be turned into an advantage. Their analysis strategy for effect de-aliasing is a precursor to what was described above. Underlying the three problems is the use of reparametrization and exploitation of non-orthogonality among some effects. The stated approach can be extended beyond designed experiments and potential applications go beyond engineering. |
09.15 - 10.45 Screening experiments and model selection (Chair: Byran Smucker) |
Brad Jones (SAS-JMP, USA) "Powerful Model Selection for Definitive Screening Designs"
Definitive screening designs (DSDs) are a powerful new approach to screening that provide the investigator a way to learn about main effects, two-factor interactions (2FIs), and quadratic effects in a number of runs that is roughly twice the number of factors. Main effects are orthogonal to each other as well as to 2FIs and quadratic effects. However, 2FIs and quadratic effects are correlated though not confounded. Still, this correlation makes foolproof model selection difficult. This talk shows how to take advantage of the special structure of a DSD to get the most clear-cut results possible. |
Kalliopi Mylona (University of Southampton, UK) "Supersaturated Split-Plot Designs: Construction and Statistical Modelling"
In this work, we develop a new general methodology for setting up and analyzing informative experiments with both restricted randomisation and a large number of factors. As designs with restricted randomisation are often much more cost-efficient than completely randomised designs, they are extremely popular in industry for quality improvement experiments and for experimenting with new products or processes. In addition supersaturated designs (SSDs) is a large class of factorial designs which can be used for screening out the important factors from a large set of potentially active variables. The huge advantage of these designs is that they reduce the experimental cost drastically but their critical disadvantage is the confounding involved in the statistical analysis. We introduce a new Bayesian optimal design criterion that can be applied to the construction of this class of designs. For model selection, we combine ideas from Bayesian inference, REML and shrinkage regression to identify important factorial effects. |
Frederick Phoa (Statistica Sinica, Taiwan) "Construction of efficient designs with circulant property and its application in fMRI experiments"
An experimental plan with circulant property is desired in many real-life experiments, like functional magnetic resonance imaging (fMRI) experiments, but it is unfortunate that these designs are lack of systematic construction approach. This talk proposes a new construction method of highly efficient designs via complete difference sets (CDS), which is a generalization of a useful mathematical tool called difference sets. The resulting classes of designs include circulant partial Hadamard matrices (CPHM) in two-levels and circulant almost orthogonal arrays (CAOA) in high-levels. The equiva- lence relation of CDS and its corresponding designs is proved. The CAOA of any prime power symbols are also constructed. This method is further applied to planning the fMRI experiments. |
10.45 - 11.15 Coffee |
11.15 - 12.45 Contributed I (Harris room) (Chair: Dibyen Majumdar) |
Shih-Hao Huang (Academia Sinica, Taiwan) "Optimal group testing designs for estimating prevalence with uncertain testing errors"
We investigate optimal designs for group testing experiments in which the goal is to estimate an unknown prevalence, and where the sensitivity and specificity of the individual tests are either unknown, or are known but are less than 100%. In the setting of known but imperfect sensitivity and/or specificity, a design with a single group size is optimal, but if the sensitivity or specificity is unknown, the optimal design has three different group sizes occurring with equal frequency. In an alternative framework in which the design must be optimal for the prevalence but not necessarily for estimating unknown sensitivity or specificity values, then a thee-point design continues to be optimal, but with unequal group size frequencies. Our work provides insight into the differing consequences of reduced sensitivity and reduced specificity on estimation performance in a group testing setting. |
Vasiliki Koutra (University of Southampton, UK) "Designing experiments on social networks"
More and more social systems can be modelled and visualised as social networks (e.g. co-authorship, friendship, online social networks etc). This highlights the practical importance of designing experiments on social networks. In this talk, we investigate the impact of the network structure on the experimental design. It seems that the experimental design is determined by the small-scale properties of networks. We discuss how to speed up the search for finding the optimum designs by utilizing the network symmetry as defined through the automorphism group of the underlying graph. Moreover, we suggest a technical framework for finding optimum block designs, while taking into account the interrelations of groups of people within the social network. In doing so, the experimental units are initially divided into blocks using spectral clustering techniques and the concept of modularity, prior to assigning the treatments. Throughout the talk, we provide several optimum designs on social networks to best estimate the direct and indirect treatment effects as found using a simple exchange algorithm, which is implemented in R. |
Ben Parker (University of Southampton, UK) "Optimal design of experiments on connected units"
Using examples from social networks, we investigate how connections between experimental units affect the design of experiments on those experimental units. Specifically, where we have unstructured treatments, whose effect propagates according to a novel linear network effects model which we introduce, we show that optimal designs are no longer necessarily balanced; we further demonstrate how experiments which do not take a network effect into account can lead to much higher variance than necessary and/or a large bias. We demonstrate how this methodology can apply in a very wide range of experiments in agricultural trials, and crossover trials, as well as experiments on connected individuals in a social network. We provide an abstraction between this class of experiments, and an experiment on a network, and use this abstraction to design algorithms which enable faster optimal design for many experiments. We expand our simple model to show how this work can be of more general appeal. |
Mahasen Dehideniya (Queensland University of Technology, Australia) "Bayesian experimental design for discriminating between models with intractable likelihoods"
In many real world applications in areas such as epidemiology and biology, the likelihood of the probability model cannot be evaluated analytically or is computationally infeasible to evaluate a large number of times. This creates an extra challenge in designing experiments to collect data in these areas. In this work, we propose a methodology for deriving Bayesian experimental designs for discriminating between rival models with computationally intractable likelihoods. Our approach follows recent research in Bayesian experimental design where approximate Bayesian computation (ABC) is used for inference such that likelihood evaluations are avoided. For the utility function, we propose using the mutual information for model discrimination which can be estimated efficiently via the ABC rejection method based on a collection of pre-simulated data. As searching for optimal Bayesian experimental designs can be a computationally demanding task, we propose a novel adaptation of the Exchange algorithm which exploits parallel computational architectures. The proposed optimization algorithm explores the design space by iteratively refining the search space around the local optimal design found in the previous iteration. This work is motivated by applications of experimental design in discriminating Markov process models in epidemiology. |
Satya Prakash Singh (Indian Institute of Technology Bombay, India) "Optimal two-treatment crossover designs for binary response models"
Optimal two-treatment, $p$ period crossover designs for binary responses are determined. The optimal designs are obtained by minimizing the variance of the treatment contrast estimator over all possible allocations of $n$ subjects to $2^p$ possible treatment sequences. An appropriate logistic regression model is postulated and the within subject covariances are modeled through a working correlation matrix. The marginal mean of the binary responses are fitted using generalized estimating equations. The efficiencies of some crossover designs for $p=2,3,4$ periods are calculated. The effect of misspecified working correlation matrix on design efficiency is also studied. |
11.15 - 12.45 Contributed II (James room) (Chair: Kalliopi Mylona ) |
Ying Hung (Rutgers University, USA) "Optimizing Latin hypercube designs by particle swarm"
Latin hypercube designs (LHDs) are widely used in many applications. As the number of design points or factors becomes large, the total number of LHDs grows exponentially. The large number of feasible designs makes the search for optimal LHDs a difficult discrete optimization problem. To tackle this problem, we propose a new population-based algorithm named LaPSO that is adapted from the standard particle swarm optimization (PSO) and customized for LHD. Moreover, we accelerate LaPSO via a graphic processing unit (GPU). According to extensive comparisons, the proposed LaPSO is more stable than existing approaches and is capable of improving known results. |
Olga Egorova (University of Southampton, UK) "Optimality criteria for multiple objectives accounting for model misspecification"
We consider the framework of a factorial experiment with a relatively small number of runs, fitted polynomial regression model and focus on developing criteria that would comprise the goodness of the fitted model parameters’ estimations, take into account the potential lack of fit of the model and minimise the mean square error from the possible model misspecification. First we adapt the Generalised A- and D-criteria (Goos et al., 2005) by treating the components from a “pure error” perspective (Gilmour and Trinca, 2012) and come up with Generalised LP- and DP- criteria. We considered two examples which displayed no definite relationship between the weights allocated to the components of the criteria and the resulting performances of the optimal designs, but in general illustrated the necessity of putting weights on the components corresponding to the desirable properties. Then instead of the predicted bias component we consider the expectation of the MSE taken over the prior for the potential terms as a well-interpretable measure of potential model misspecification. One of the noteworthy observations here is that a certain contradiction between the DP- (LP-) and MSE-components of the criteria occurs, which for now means that it would be difficult to find a compromise between precise estimations of the primary model parameters and minimise the deviation of the estimates in case the model is very likely to be incorrect. The point exchange algorithm was implemented in order to conduct the search for (nearly) optimal designs; some additional computational features are also discussed. |
Kim May Lee (University of Southampton, UK) "Optimal design for linear models with responses missing at random"
In statistical studies, having missing values in the collected data sets is often unavoidable. Most of the work done in the area of experimental design assumed that responses are always fully observed. Experimenters rarely consider the impact of missing values in the collected data, which can occur in the factor, response, or all the variables of the experiment. Following Imhof, Song and Wong's (2002), we consider the missing data analysis methods at the design stage of an experiment. Considering complete case analysis for the responses missing at random, an optimal design framework for the general linear model will be introduced. We also consider a multiple imputation approach in the design context. We show that the optimal designs found by using the framework with complete case analysis is sufficient for the experiment with responses missing at random. The performance of the different optimal designs will be compared by some simulation studies for a simple linear model. |
Varun Khemani (University of Maryland, USA) "Power and practicality of large supersaturated designs"
The most frequently studied supersaturated design in the literature is the 23 factor 14 run design. However, there has been a dearth in literature on the analysis of large supersaturated designs, which are considered by many to be impractical. Analysis of small supersaturated designs analysis even poses significant intricacies and established methods when applied to large supersaturated designs tend to break down. We intend to shift the focus in literature to large supersaturated design analysis while simultaneously investigating the effect of specific violations of the effect sparsity principle. We use a 125 factor 24 run Es2 optimal balanced design by acquiring data from the additive manufacturing domain. We also introduce several methods existing in literature but never applied to supersaturated designs. These methods include Bayesian Compressive Sensing, Bayesian Horseshoe prior, algorithms for constrained optimization and unconstrained optimization. These methods in general provide better performance than the current analysis methods. |
Roselinde Kessels (University of Antwerp, Belgium) "Practical optimal design of discrete choice experiments with partial profiles"
One objective of many discrete choice experiments (DCEs) is to investigate people’s preferences for a large number of attributes of a product or program under study for improvement. To bridge the gap between the cognitive load in choosing between competing options or profiles, and the incorporation of many attributes in these profiles, we recommend using partial profile designs. In these designs, the levels of some attributes are held constant within a choice situation. To design a DCE with partial profiles, it is necessary to make two important decisions for each choice situation. First, the set of attributes the levels of which are held constant has to be determined. Second, the levels of the varying attributes have to be selected. This should be done with the objective of maximizing the quality of the information produced by the experiment in such a way that the underlying discrete choice model can be estimated as precisely as possible. In this presentation, we lay out the pros and cons of using analytical and algorithmic approaches for the generation of optimal partial profile designs for both main-effects models and models with interaction effects. We consider our newly developed two-stage and integrated algorithms for the generation of utility-neutral, locally optimal and Bayesian optimal designs. By means of extensive computational experiments, we compare computing times and the statistical efficiency and structure of the designs obtained. We end with an application of a DCE to prioritize various health care interventions for which we constructed a Bayesian optimal partial profile design for the estimation of an interaction-effects model. |
12.45 - 13.45 Lunch |
13.45 - 14.45 Bayesian design (Chair: Antony Overstall) |
Maria Adamou (University of Southampton, UK) "Bayesian optimal design for Gaussian process regression"
Data collected from correlated processes arise in many diverse application areas including both computer and physical experiments, and studies in environmental and ecological science. Often, such data are used for prediction and optimisation of the process under study. For example, we may wish to construct an emulator of a computationally expensive computer model, or simulator, and then use this emulator to find settings of the controllable variables that maximise the predicted response. The design of the experiment from which the data are collected may strongly influence the quality of the model fit and hence the precision and accuracy of subsequent predictions and decisions. We consider Gaussian process models that are typically defined by a correlation structure that may depend upon unknown parameters. This parametric uncertainty may affect the choice of design points, and ideally should be taken into account when choosing a design. We consider Bayesian design and use a new approximation to the expected loss to find decision-theoretic optimal design for prediction. The resulting designs are illustrated through a number of simple examples typical of computer experiments and spatial statistics. |
James McGree (Queensland University of Technology, Australia) "A pseudo-marginal sequential Monte Carlo algorithm for random effects models in Bayesian sequential design"
A particle filter approach will be presented that can be used for Bayesian sequential design for random effects models in the presence of model and parameter uncertainty. Our focus is on sequential design where determining the next ‘best’ design point is defined via a utility function which could, for example, focus on parameter estimation, model discrimination or a combination of these two objectives. Particle filters are run for each rival model, and the algorithm relies on a convenient estimator of the posterior model probabilities for each model to efficiently approximate utility values. Implementing particle filters for random effects models requires evaluating the likelihood a large number of times. Unfortunately, this likelihood is generally analytically intractable for all but the simplest of models. In our work, we propose approximating the likelihood via a variety of Monte Carlo methods. Efficiency in computing these approximations is achieved via exploiting parallel computational architectures, which will be discussed. We motivate our research by the application of sequential design in the treatment of critical ill patients who are on cardiac and respiratory support. |
14.45 - 15.30 Posters and Tea |
15.30 - 16.30 Optimal design for stochastic processes (Chair: Ben Parker) |
Richard Boys (Newcastle University, UK) "Efficient construction of optimal designs for stochastic kinetic models"
Stochastic kinetic models are discrete valued continuous time Markov processes and are often used to describe biological and ecological systems. In recent years there has been interest in the construction of Bayes optimal experimental designs for these models. Unfortunately standard methods such as that by Muller (1999) are computationally intensive even for relatively simple models. However progress can be made by using a sequence of Muller algorithms, where each one has an increasing power of the expected utility function as its marginal distribution. At each stage efficient proposals in the design dimension can be made using the results from the previous stages. In this talk we outline this algorithm, investigate some computational efficiency gains made using parallel computing and illustrate the results with an example. |
Joshua Ross (University of Adelaide, Australia) "Design of group dose-response challenge experiments"
Dose-response challenge experiments are used extensively to define safe levels of certain bacterium, and assess the quality or risks associated with a particular drug. Our interest will be in estimating the dose-response relationship of Campylobacter jejuni in chickens. A complication to this assessment is that chickens are social animals -- hence ethically must be housed in groups -- and engage in coprophagy; a consequence being that transmission of infection may occur via the faecal-oral route. Furthermore, the accurate evaluation of infection of a chicken requires its sacrifice. Hence questions of design naturally arise: (i) how many chickens should one have in each group?; (ii) how many groups should one have?; (iii) what doses should one give the groups?; and, (iv) at what times should one sacrifice the chickens? We will answer these questions by developing appropriate Markovian models and using Approximate Bayesian Computation to identify the optimal design. Consideration of the impact of choice of utility will be highlighted and discussed. |
Day 3: Wednesday 16 December 2015 |
08.15 - 08.30 Registration |
08.30 - 09.15 Keynote (Chair: Min Yang) |
Ping Ma (University of Georgia, USA) "Leveraging in big data regression"
Rapid advance in science and technology in the past decade brings an extraordinary amount of data, offering researchers an unprecedented opportunity to tackle complex research challenges. The opportunity, however, has not yet been fully utilized, because effective and efficient statistical tools for analyzing super-large dataset are still lacking. One major challenge is that the advance of computing resources still lags far behind the exponential growth of database. To facilitate scientific discoveries using current computing resources, one may use an emerging family of statistical methods, called leveraging. Leveraging methods are designed under a subsampling framework, in which one samples a small proportion of the data (subsample) from the full sample, and then performs intended computations for the full sample using the small subsample as a surrogate. The key of the success of the leveraging methods is to construct nonuniform sampling probabilities so that influential data points are sampled with high probabilities. These methods stand as the very unique development of their type in big data analytics and allow pervasive access to massive amounts of information without resorting to high performance computing and cloud computing. In this talk, I will present some leveraging estimators in big data regression and their associated statistical properties. |
09.15 - 10.45 Design for clinical and pharmaceutical applications (Chair: Tim Waite) |
Lisa Hampson (Lancaster University, UK) "Optimal group sequential tests for delayed responses with non-binding futility boundaries"
Group sequential tests propose monitoring data as they accumulate from a clinical trial rather than waiting until the end of a study to make a final decision. One-sided group sequential tests are usually designed assuming that recommendations to stop a trial early, for efficacy or lack of benefit, will always be adhered to. Unplanned deviations from a binding futility rule will cause inflation of the type I error rate. However, a sponsor may wish to have the flexibility to continue a trial even after a futility boundary has been crossed in order to gather additional data on safety or key secondary endpoints. In this presentation we formulate group sequential designs for delayed responses with non-binding futility rules. Delayed responses are common in practice since clinical interest often lies in testing the long-term effects of a new treatment on patients’ health. We seek tests which control the type II error rate when early stopping recommendations are always followed, and control the type I error rate when futility boundaries are always disregarded. We use dynamic programming to find optimal versions of designs satisfying these frequentist error rate constraints as solutions to Bayes decision problems. Properties of optimal rules are used as a benchmark to understand the relative efficiencies of competing designs specifying futility boundaries on the basis of predictive or conditional power, for example. |
Stefanie Biedermann (University of Southampton, UK) "Optimal designs for survival experiments"
Censoring occurs in many industrial or medical ‘time to event’ experiments/trials. Finding efficient designs for such experiments can be problematic for various reasons: 1. The statistical models involved may be nonlinear, making the optimal choice of design parameter dependent; 2. In addition, there may be uncertainty about the form of the model; 3. More flexible semiparametric models may be used for analysis, for which only limited theory on optimal design is available; 4. There may be an excess of instant failures, which may not be captured adequately by standard survival models, resulting in the use of more sophisticated models, e.g. mixture models. We present strategies for addressing these issues, and provide recommendations for practitioners. Our results are illustrated through a simulation study assessing the performance of the recommended designs in various scenarios of practical relevance. |
Feifang Hu (George Washington University, USA) "Adaptive designs and personalized medicine"
In decades, scientists have identified genes (biomarkers) that seem to be linked with diseases. To translate these great scientific findings into real-world products (Personalized Medicine), new approaches to the drug-development paradigm are needed. In this talk, I will discuss adaptive design of clinical trials for personalized medicine. To deal with the special features of personalized medicine, we need new families of adaptive designs to: (i) balance many important covariates; (ii) detect the interaction between treatment and biomarkers efficiently; or (iii) incorporate covariate information in adaptive designs by considering both efficiency and medical ethics. Some new designs are proposed and their properties are discussed. Advantages of the proposed methods are demonstrated through both theoretical and numerical studies. Some further statistical issues are also discussed. |
10.45 - 11.15 Coffee |
11.15 - 12.45 Design construction (Chair: Ching-Shui Cheng) |
Byran Smucker (Miami University, USA) "Generating and comparing Pareto fronts of experiment designs to simultaneously account for multiple experimental objectives"
There is a general and growing acknowledgement within the experiment design community that in realistic design scenarios the experimenter typically entertains multiple, conflicting objectives. The Pareto front approach to optimal experiment design constructs a set of designs while explicitly considering tradeoffs between opposing criteria. The true Pareto front in these optimization problems is not known, and this creates problems in assessing the quality of competing Pareto fronts. Furthermore, existing algorithms are either relatively slow, or produce low quality fronts, or both. In this talk, we present a new algorithm to generate Pareto fronts of designs, and discuss a more general framework for algorithm development in this area. We also briefly introduce an improved measure of front assessment that provides a way to fairly compare Pareto fronts. |
Radoslav Harman (Comenius University of Bratislava, Slovakia) "On convex programming methods for computing optimal designs of experiments under non-standard constraints"
Modern methods of convex optimization, such as the second-order cone programming (SOCP) and the semidefinite programming (SDP), provide powerful alternatives to the traditional, first-order algorithms for computing optimal experimental designs under non-standard experimental constraints. However, the choice of the most efficient mathematical programming method may strongly depend on the specifics of the criterion of optimality as well as on the type of the constraints. In the talk, I will give a brief survey of the current state of knowledge about the mathematical programming approach to optimal design of experiments under general linear constraints, focusing on the regression models with uncorrelated observations and finite design spaces. I will also compare the mathematical programming and traditional methods with respect to their speed, memory requirements and the scope of application. |
Stelios Georgiou (RMIT University, Australia) "Combinatorial structures and orthogonal designs for computer experiments"
Latin hypercube designs is a class of experimental designs that is of huge importance when computer simulations are needed to study a physical process. In this talk, a general method is proposed for constructing orthogonal Latin hypercube designs and designs for computer experiments. In particular, combinatorial structures, orthogonal designs (ODs), generalized orthogonal designs (GODs) and sequences with zero autocorrelation function are used to construct orthogonal Latin hypercube designs and designs for computer experiments. The generated designs have some additional favourable properties such as orthogonality of the first and some second order terms, and optimality under some evaluation criteria. |
12.45 - 13.45 Lunch |
13.45 - 14.45 Uncertainty quantification I (Chair: Jeff Wu) |
Matthew Plumlee (University of Michigan, USA) "Orthogonal Gaussian processes: Making a mean mean what it should mean"
Computer simulators invariably have, as their foundation, a mathematical representation of the natural or physical process they are attempting to describe and simulate. The long process from defining the mathematical model, finding solutions of this model, representing these solutions in a numerical procedure, then implementing and executing the procedure in computer codes, introduce uncertainties at each step. These uncertainties need to be defined, characterised, accounted for and controlled in a systematic manner to ensure that subsequent inferences made about the system under study proceed in a rational manner. This talk will review the various sources of uncertainty and their implications to the scientific process. Statistical representations of this uncertainty will be considered and some recent research work will be presented illustrating the challenge faced in characterising the cumulative effect of these uncertainties to the inference process. |
Robert Gramacy (University of Chicago, USA) "Local Gaussian process approximation for large computer experiments"
Gaussian processes models are widely adopted for use in uncertainty quantification. However, identifiability issues occur when the mean model contains low-order polynomials with unknown coefficients. This leads to poor estimation of the coefficients in the mean model. This work introduces a new Gaussian process model whose stochastic part is orthogonal to the mean part to address this issue. |
14.45 - 15.00 Tea |
15.00 - 16.00 Optimal design (Chair: Peter Goos) |
Dibyen Majumdar (University of Ilinois at Chicago, USA) "Optimal supersaturated designs"
We consider screening experiments where an investigator wishes to study many factors using fewer observations with two level factors and a main effects model with intercept. We introduce and study $UE(s^{2})-$optimality, which is essentially the same as $E(s^{2})-$optimality, except that we do not insist on factor balance. We also introduce a second criterion, D-optimal supersaturated designs, that is based on the minimum bias estimator. We provide a simple construction of D-optimal supersaturated designs using D-optimal chemical balance weighing designs obtained by Galil and Kiefer (1980, 1982), Cheng (1980) and other authors. It turns out that, except when the number of observations and the number of factors are in a certain range, an $UE(s^{2})-$optimal design is also a D-optimal supersaturated design. Moreover, these designs have interesting connections with Bayes optimal designs. When the prior variance is large enough, a $D-$%optimal supersaturated design is Bayes $D-$optimal and when the prior variance is small enough, an $UE\left( s^{2}\right) -$optimal design is Bayes $D-$optimal. While $E(s^{2})-$optimal designs yield more precise intercept estimates, our study indicates that $UE(s^{2})-$optimal designs generally produce more efficient estimates for the factors. |
Ching-Shui Cheng (Academia Sinica, Taiwan, and University of California at Berkeley, USA) "Optimal designs for quadratic regression with random block effects: the case of block size two"
Optimal approximate designs for quadratic regression with random block effects in the case of block size two are considered. We obtain, with respect to the Schur ordering, an essentially complete class consisting of designs with a simple structure. The locally D- and A-optimal designs given in Cheng (1995) and Atkins and Cheng (1999) belong to this class. We explicitly identify locally E-optimal designs and show that for each $p, -\infty\leq p\leq 1$, there is a unique $\phi_p$-optimal design in this class. Bayesian $\phi_p$-optimal designs are also considered. |
Day 4: Thursday 17 December 2015 |
08.30 - 09.15 Keynote (Chair: Debbie Street) |
Kathy Ruggeiro (University of Auckland, New Zealand) "Optimal block designs in the absence of unit-treatment additivity"
Classically optimal designs are generated by assuming unit-treatment additivity, i.e. functional independence between observations' means and their variances and covariances. Responses in many modern experiments, however, are drawn from distributions such as the one and two parameter exponential families, e.g., RNA sequence counts from a negative binomial distribution. These violate additivity. Work has been done on generating optimal designs for general exponential family distributions in the context of response surface models where the values of the treatment factor levels are allowed to vary to achieve optimality. We consider, in contrast, block designs for the class of designs in which the values of the factor levels are fixed at the outset and play no role in design optimality. We will show that optimal designs from the classical setting can be importantly non-optimal when additivity is violated and present an approach for generating optimal block designs when the observations follow a Poisson distribution. |
09.15 - 10.45 Uncertainty quantification II (Chair: James McGree) |
Rui Tuo (Chinese Academy of Sciences, China) "Efficient calibration for imperfect computer models"
Many computer models contain unknown parameters which need to be estimated using physical observations. Tuo and Wu (2014) shows that the calibration method based on Gaussian process models proposed by Kennedy and O’Hagan (2001) may lead to unreasonable estimate for imperfect computer models. In this work, we extend their study to calibration problems with stochastic physical data. We propose a novel method, called the L2 calibration, and show its semiparametric efficiency. The conventional method of the ordinary least squares is also studied. Theoretical analysis shows that it is consistent but not efficient. Numerical examples show that the proposed method outperforms the existing ones. |
Xu He (Chinese Academy of Sciences, China) "A constructive method for generating near optimal minimax distance designs"
We propose a new class of space-filling designs called rotated sphere packing designs. The approach starts from the asymptotically optimal positioning of identical balls that covers the unit cube. Properly scaled, rotated, translated and extracted, such designs are near optimal in minimax distance criterion, low in discrepancy, good in projective uniformity and thus useful in both prediction and numerical integration purposes. The proposed designs can be constructed for any given numbers of dimensions and points. |
Matthias Tan (City University, Hong Kong) "Monotonic quantile regression with Bernstein polynomials for stochastic simulation"
Quantile regression is an important tool to determine the quality level of service, product, and operation systems via stochastic simulation. It is frequently known that the quantiles of the output distribution are monotonic functions of certain inputs to the simulation model. Thus, it can be valuable to incorporate this information in quantile modeling. In this paper, we propose a class of monotonic regression models, which consists of functional ANOVA decomposition components modeled with Bernstein polynomial bases, for estimating quantiles as a function of multiple inputs. The polynomial degrees of the bases for the model and the FANOVA components included in the model are selected by a minimizing the Bayesian information criterion. Real examples demonstrate the good performance of the proposed methodology for estimating quantiles. |
10.45 - 11.15 Coffee |
11.15 - 12.45 Design for linear models (Chair: Steve Bush) |
JP Morgan (Virginia Tech., USA) "Fractions, potential terms, weighting, and relative efficiency"
DuMouchel and Jones (1994) introduced an approach to D-optimal design selection allowing estimation of all primary terms (those in the assumed model) with good detectability for potential terms (terms which may or may not be contributors to the actual data generating mechanism). An asymptotic examination of their modified information matrix establishes a connection with a corresponding weighted information matrix. Combining the two techniques provides another avenue for generating useful fractional factorial designs. The role of symmetry in the optimality argument is also examined for its implications on design efficiency measures. |
Wei Zheng (Indiana University-Purdue University Indianapolis, USA) "Universally optimal circular designs for the interference model"
In many blocks designs, a treatment targeted on a particular unit inevitably interferes the response on its neighbour units due to the spatial or temporal structure of the block. Recently, Zheng (2015) provided equivalent conditions for any design to be universally optimal in approximate design theory. Unfortunately, the method therein can not be applied to the important circular setup where where each block has a guard plot at each end. Optimal designs under this setup has been studied in the last two decades. Many designs has been proposed and their efficiencies are studied under different conditions. The drawback of this approach is that the proposed designs only exist under rare cases. Here, we try to deliver a unified framework for deriving optimal or efficient designs under all possible conditions. The produced designs will be at least as efficient as the particular proposed structure when they exist. Technically speaking, Zheng (2015)'s method heavily rely on the positive definiteness of a Hessian matrix, which can no longer hold under the circular setup. This is the first work dealing with singular Hessian matrix and shed light on other designs problems with the same difficulty, mostly due to the circular setup. |
Jon Stallings (North Carolina State University) "Carryover designs including washout periods"
Carryover designs (or crossover designs) are frequently used when subjects are assigned multiple treatments across sequential periods. Different treatments are often applied with little time between them, potentially leading to residual treatment effects in subsequent periods. One way to eliminate the residual effect is to include a washout period, during which no treatment is assigned to the subject. However, this increases the overall time required for the experiment to be conducted. Because of this, most research on carryover designs ignores including washout periods and instead constructs designs based on a statistical model incorporating the residual effects. Here I consider scenarios in which some, but not all, treatments may be followed by a washout period. I detail the possible modifications to the usual carryover model and highlight their impacts on optimal designs for estimation of direct and/or residual treatment effects. Optimal designs are derived for specific cases of the number of subjects, periods, and treatments. For more complicated scenarios, an exchange algorithm is run and properties of the optimal designs are investigated. |